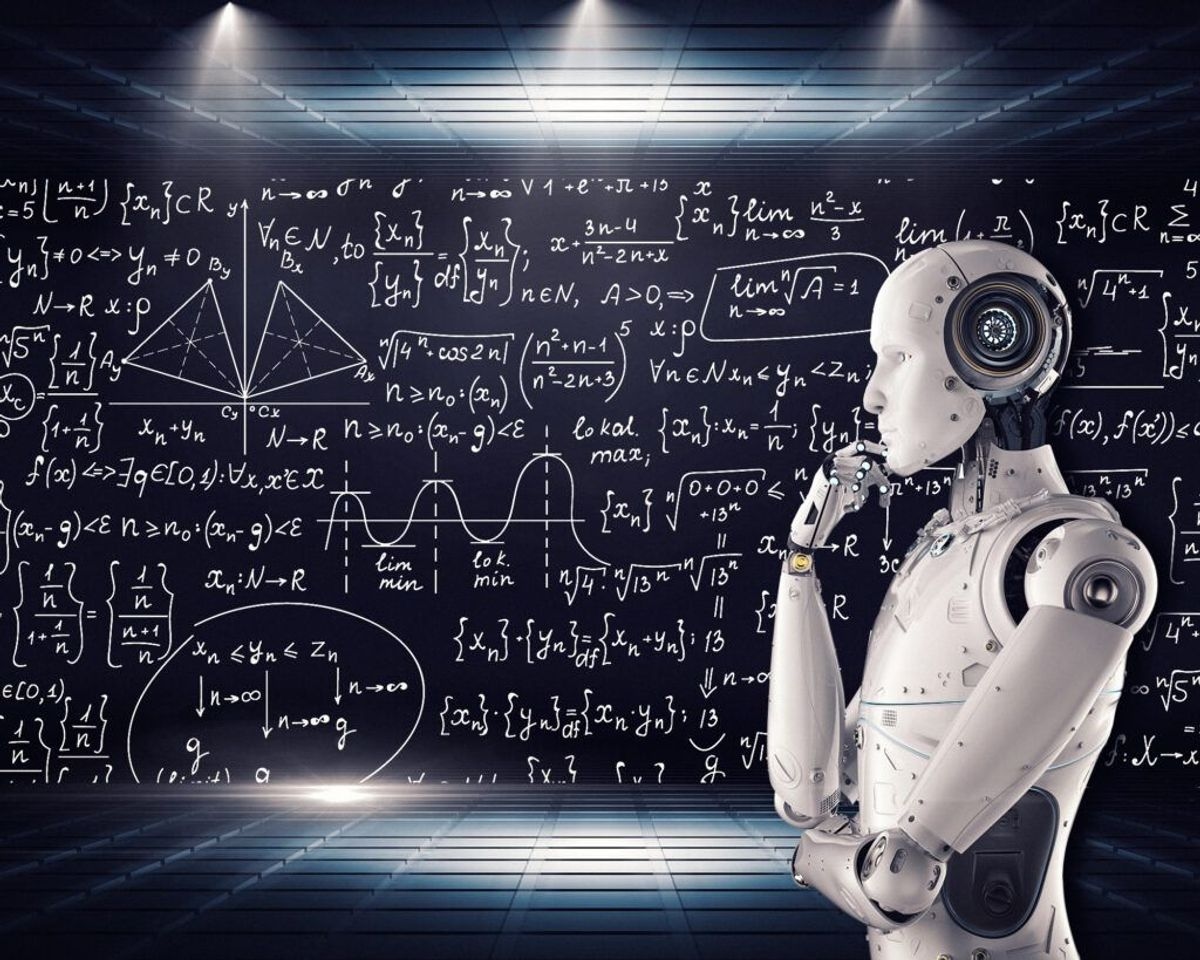
Claims of AI achievements need proper assessment
Image courtesy of mikemacmarketing/www.vpnsrus.com
Google recently released a new iteration of its AI large language model PaLM2.
To demonstrate its capabilities, developers had it 'sit' advanced language assessments in German, Italian, Spanish, French, Chinese and Japanese.
It passed all at ‘mastery’ level.
That's an impressive result, on the surface. But the fine detail gave me concern about the way AI systems’ performances are being assessed and portrayed.
Did PaLM2 actually pass a language test?
The technical report shows PaLM2 passed part of a recognised test using a changed scoring system, marked by non-experts and awarded a sometimes dubious percent score.
To explain my reasoning, let’s drill down into PaLM2’s performance using the PLIDA Italian language proficiency test it sat as an example.
Firstly, PLIDA assesses Reading, Listening, Writing and Speaking.
PaLM2 cannot be assessed on Speaking, and the transcript of the Listening component was used as a Reading test.
That means it did not sit the whole test and casts doubt over the ’overall’ assessment.
How was PaLM2's language assessment marked?
Now we come to the scoring system.
PLIDA scores each of its components out of 30 while PaLM2’s Writing was scored out of 5.
The only question asked of the markers was something like ‘does this read like a native speaker wrote it?’
Imagine you’re a marker and think PaLM2 did ‘OK’. Does 3 out of 5 seem right? How about 16 out of 30 using PILDA’s system?
In the first case with little room for nuanced marking , PaLM2 passes with 60%.
For the second, marked with more subtlety, 16 out of 30 is 53% and a fail.
This is why assessment scoring systems matter.
Who marked PaLM2's work?
Then there’s the humans marking it.
‘Non expert’ native speakers assessed the written test. They were not described as assessment experts qualified to rate others’ performances.
Professional markers have to make sense of marking concepts such as ‘adequacy of the register to the situational context.’ This is what they are trained to do.
It is not clear the non-experts looking at PaLM2's responses were asked, or able, to do this.
Scoring PaLM2 does not always add up
Finally, we’ll move on to the scores I described as ‘sometimes dubious.’
Three people marked the Writing out of 5 and the average was used to calculate a percentage.
For Chinese and French, PaLM2 scored an impressive 82% and 85% respectively.
However, these percentages are unachievable from an average of three scores out of 5. Even considering rounding error, there’s a jump from 80% to 87% ruling out any score in between.
These are small errors, but how many others may there be?
Was there a lack of assessment know-how applied to PaLM2?
I may be accused of being unreasonably picky. Sure, these are unofficial results to demonstrate PaLM2’s capabilities but, the way Google casually rebuilt a well thought out, rigorous assessment reveals a worrying issue – the lack of assessment literacy within the AI community.
Quite often, developers have no understanding of assessment and may not realise it is a standalone discipline.
Whenever they train, assess and evaluate their systems, they do so, not as experts in assessment but in AI development.
This matters because these systems are increasingly required to perform tasks alongside or in place of people.
Imagine an AI model as a student being trained to do something – you’d want the training delivered by an expert in the subject and pedagogy.
Assessment, likewise, should also be carried out by an expert.
AI should be evaluated with the same rigour applied to humans on those same tasks.
There shouldn’t be free passes simply because no comparable assessments for AI exist.
If PaLM2 can act like a human should it be assessed as one?
The more we conceptualise AI in human terms, the greater the need is to bridge machine learning with human learning using assessment experts.
There’s room for optimism however. OpenAI had qualified markers look at written assessments its GPT-4 model created.
I hope collaboration like this between assessment experts and AI professionals continues to grow and furthers research in the field.
While I have shown why I think PaLM2 didn’t actually pass language tests, knowing whether it could pass a proper, real-world language test could have important repercussions on how we assess people.
Read More By This Author:
AI and Exam Marking - trust and accountability
AI and Reviews of Marking - how is the future looking
Validity and Trust in Algorithms for Assessment and Scoring
Read More On This Topic:
If Exams Stay Don't Fear ChatGPT
Adaptive Assessment - tailoring the future
Can Digital Technology Transform Assessment Practices